Streamline Modeling
Streamline-based flow simulation for the purpose of ranking large-scale geologic realizations continues to receive significant attention. However, the procedures and the analyses for ranking are not straightforward and therefore actual case examples are very limited.
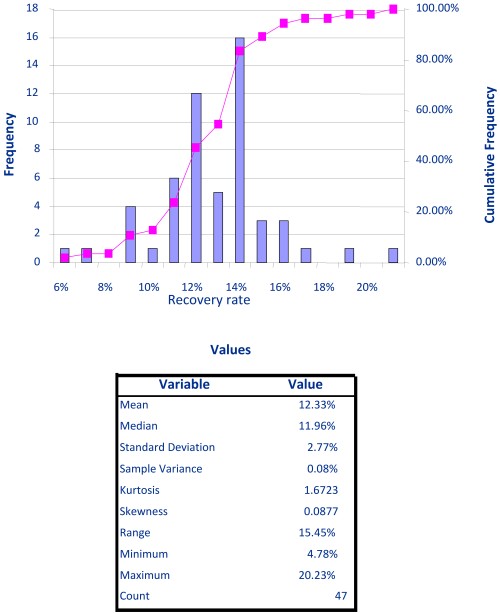
Figure 1 : Conventional view of uncertainty analysis from an engineering perspective — reducing complex 3D simulation results into a recovery/ rate uncertainty range for economic calculations.
Anyone involved in reservoir simulation realizes there are several potential sources of errors or uncertainties when doing a reservoir study:
- Numerical error (from the approximate solution of non-linear partial differential equations);
- Error from the approximations in the underlying equations (e.g. 3-phase approximation of Darcy’s law);
- Errors or uncertainties in data interpretation (e.g. converting log signals to reservoir properties);
- Ignored data (e.g. not using the seismic data in reservoir property distribution);
- Unknown or uncertain data (e.g. only a small portion of the reservoir is sampled); and
- Incorrect averaging of data (e.g. averaging log measurements over a flow unit).
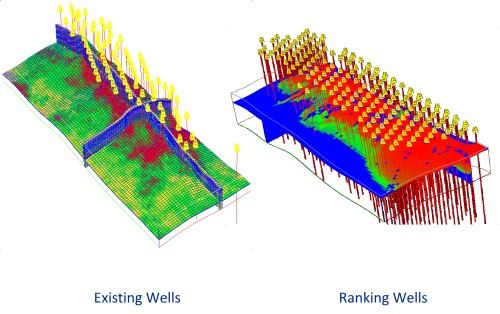
Figure 2 : Uniform injection production patterns used over the entire area of interest allow an understanding of the geologic variability of the realizations.
This work demonstrates a methodology for ranking and analyzing the impact of geologic uncertainty on future recovery from a mature field in the continental U.S using a streamline simulator. Multiple fine-grid realizations were developed with a commercial geo-modeling software program and these realizations were evaluated with a commercial streamline simulator. The results from these simulations were then used to evaluate the recovery uncertainty and to rank these realizations. Several of these realizations were then used in a conventional commercial simulator to compare recovery differences for more rigorous evaluations.
The basic idea introduced in this study is to use regular well patterns in streamline simulations to study the geologic variability of the realizations. This is different from the common approach of trying to use existing wells with pseudo boundary conditions. These uniform patterns are not necessarily representative of actual well patterns or injected fluids rates. The waterflood efficiency, however, can still be used as a basis of comparison for ranking different realizations. The uniform spacing ensures complete coverage of the area-of-interest and not just the areas where the model is already conditioned to existing data. The purpose is to test “connectivity” of geologic models and to provide a graphical basis for calculating the uncertainty in recoveries as determined from the ranking process. The models are already conditioned to existing well data. This method tests the variability of the models away from existing wells as these areas will have longer-term effect on performance and affect the decision regarding future infill wells and recovery methods.
Highlights
- Uncertainty analysis means more than generating a range of field recoveries / rates for corporate risk analysis
- Streamlines are an effective method for quantifying the geologic uncertainty in large 3D geomodels
- Simple ranking based on total field recovery, water-flood efficiency, etc can be misleading
- The use of streamline simulations with uniform well patterns, statistical analysis and visualization provides an improved understanding of the effect of geologic uncertainty and can reduce risk in field projects
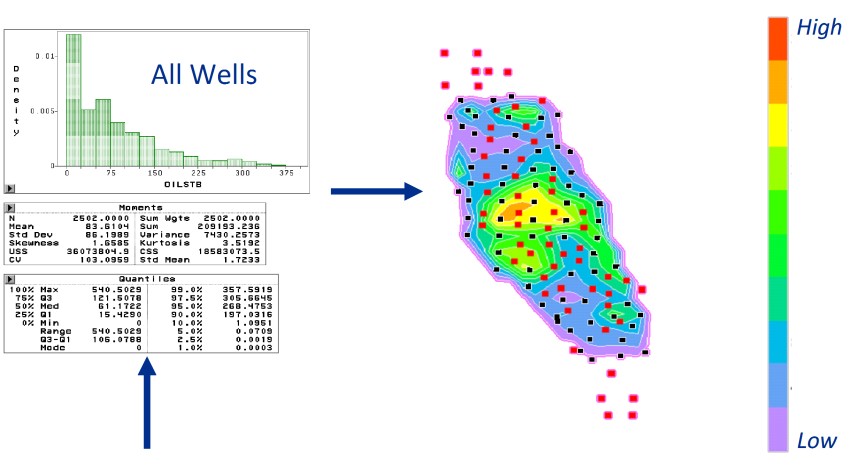
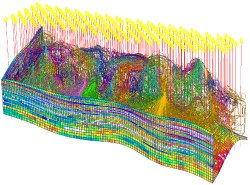
Figure 3 : Oil Recovery from one Realization with Existing Wells
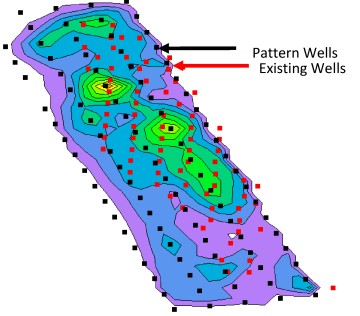
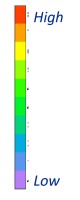
Figure 4 : Average recovery for a series of twenty-six realizations with uniform well patterns
Modern tools and analysis techniques are greatly improving the ability to understand complex reservoirs, which allows improved decisions regarding optimum development. However, with the large volume of data that is becoming available and included in the analyses, it is important to fully use the capabilities of modern software and hardware to analyze and visualize the data. This will ensure a better understanding of the interpretations and the resulting uncertainties. Loading all the simulations in a statistical program that allows rapid data analysis and is capable of handling large data sets, and generation of statistical variables is an important aspect of the methodology.
For more information: “Statistical Ranking of Stochastic Geomodels Using Streamline Simulation: A Field Application”, James R. Gilman, Hai-Zui Meng, Michael J. Uland, Peter J. Dzurman, Stevan Cosic. SPE 77374.